How can artificial intelligence help give early warning to policymakers of a conflict brewing in a region? Does machine learning perform better than the models based on theoretical understanding of instabilities? These are the questions that Hannes Mueller and Christopher Rauh try to address in their BSE Working Paper (No.990) “Reading between the Lines: Prediction of Political Violence Using Newspaper Text.”
Predicting the onset of conflicts: an arduous challenge
The conflict literature has made significant progress in understanding which countries are more at risk of suffering an armed conflict. Many factors that have been identified as leading to increased risk, like mountainous terrain or ethnic polarization, are time-invariant or very slow moving, and therefore not useful in predicting the timing of conflict. Mueller and Rauh note that the literature has been criticized for failing to provide early warning when new instabilities emerge. For example, back in 2010 none of the eagerly tracked indices of political instability were predicting a wide-ranging conflict frothing in the Middle East. This has led to some soul-searching in the literature and, most recently, to claims that forecasting new civil wars might have reached a limit.
According to authors, the struggle to forecast instabilities accurately may point to methodological shortcomings in the current literature which in some cases, attach disproportionate risk to countries that have seen a conflict in the recent past. Consequently, new onsets in formerly stable countries are very hard to predict using these methods. In other cases, the emphasis is just on within country social and political dynamics which tend to over-predict events of conflict by a wide margin. In this paper, Mueller and Rauh provide a new methodology to predict armed conflict by using newspaper text. They use the latest advances in machine learning to transform vast quantities of newspaper text into interpretable topics which provide greater forecasting ability to the model.
It’s there in the papers!
To undertake their study, the authors collect articles from three major newspapers published in English, namely The Economist (available from 1975), the New York Times (NYT) (available from 1980), and the Washington Post (WP) (available from 1977). In total, the data consists of more than 700,000 articles, of which 174,450 are from the Economist, 363,275 from the NYT, and 185,523 from the WP. On average about 120 articles are written on a country in a given year. Figure 1 below shows the time series of the number of articles downloaded from the three newspapers from 1980 to 2015. It can be seen that New York Times had the greatest coverage during the period under study.
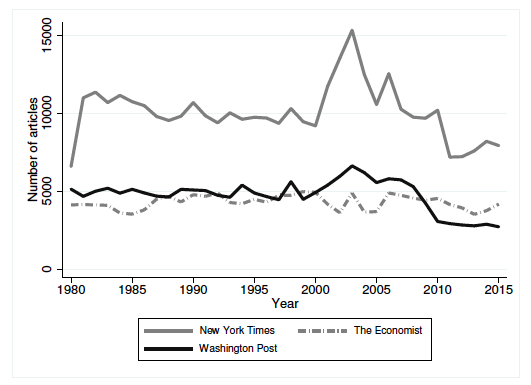
Topic model
The authors use the Latent Dirichlet Allocation (LDA) model to summarize the articles to topics that can be attributed to each article. Figure 2 below shows how words found in the articles are associated with each topic, a larger font of a word indicates a larger association with a particular topic. The relevant topics are automatically selected by the algorithm, letting the data speak for itself.
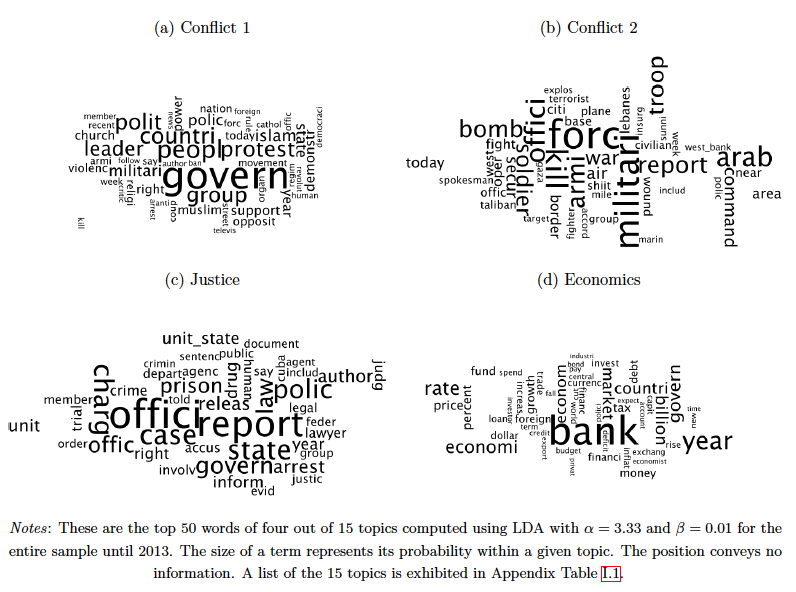
An important conceptual contribution of this paper is that Mueller and Rauh explicitly separate the within-country from the between-country variation before forecasting. This allows Mueller and Rauh to use the within-country variation, i.e. the emergence and disappearance of topics on the country level, to predict conflict out-of-sample. They show that reporting on specific topics, like conflict, increases before a conflict, whereas reporting on other specific topics, like legal procedures or economics, decreases. In this way, the timing of conflict can be forecasted more accurately than through variables previously used by the literature.
Figure 3 below shows the result of a model including only 15 topics out of 0.9 million tokens generated by LDA model from the newspapers’ articles. The model does particularly well at predicting the onset of both civil war and armed conflict. When predicting civil war onset, the news model generates a TPR, i.e. rate of predicting a conflict correctly, of about 80 percent and a FPR, which is the rate of over-predicting a conflict, of 20 percent. Furthermore, the predictive power of the within model is very close to the predictive power of the overall model. This is quite a striking finding given the difficulty of forecasting the timing of such rare events.
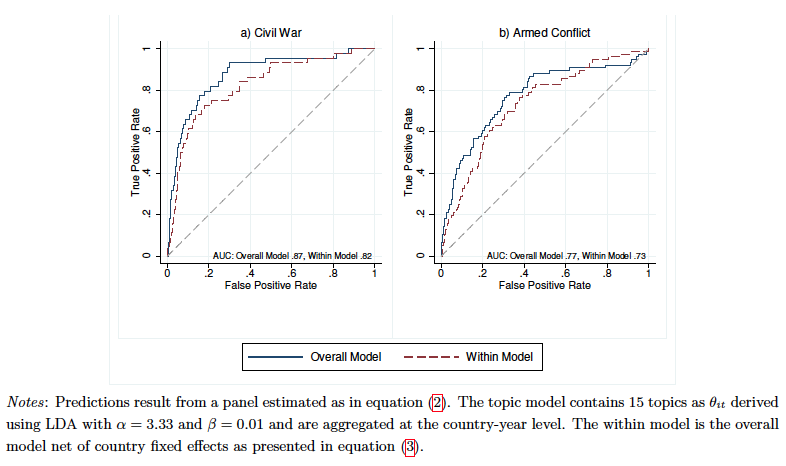
Conclusion
Mueller and Rauh present a new method of predicting conflict through news topics which are generated automatically from a topic model. They show that relying on the overall variation of models, even if they contain useful within variation, can lead to a bias against onsets in previously peaceful countries. Ultimately, researchers and policymakers therefore face a trade-off between a better prediction overall and a model that is more useful in spotting new instabilities. However, the authors are quick to note that forecasts do not provide a causal analysis of the underlying factors of high risk but only produce a warning of that risk. Additional analysis of the specific circumstances is needed to identify ways to address the conflict risk.